Let’s Do Some Engineering Pt. 2: Software Metrics
View part 1 of this series: Design Patterns.
Taken from andysartwork.com.
Software metrics don’t get their dues. A lot of us use metrics often, but we often don’t pay attention and realize why or how we’re using them. This means we’re missing a great opportunity to learn about the metrics we use, why, when and where they apply, and potentially even discover some new metrics that might be more helpful for keeping track of our code. Hopefully this article can shed some light on these metrics we use and explain when and where they’re best applied as well as when and where they aren’t. I’d also like to cover some other metrics that aren’t as popular but might be interesting to know about, especially for dynamic languages such as Python or Ruby.
To be fair there are a lot of kinds of metrics, so saying “metrics don’t get their dues” is sort of like saying “music doesn’t get respect”. There are performance metrics, runtime metrics, organizational metrics, even metrics that have little to do with code (effort estimation, which I’ll be talking about a little later in this series). There are already plenty of tools that do performance metrics, and a lot of smart people who focus on profiling and benchmarking applications. That topic seems to get a lot of attention and “respect”. I’m instead going to focus mainly on static code metrics; anything dealing with uncompiled, non-running source code.
Should I Really Care About Metrics?
Static source metrics provide you with a good sense of the overall state of your codebase at a specific time. This helps you keep track of and manage your code, but more importantly it helps you decide what parts of your code need work, either via refactoring or extra testing. Note that we touched on tracking. I’ll be covering some engineering practices relating to tracking the state of your system in another article (when I talk about estimation). For now we can assume (and we generally know) that keeping track of your code’s history is a good thing; that’s why you’re using source control, right? Metrics give us the numbers to track in our codebase. This lets us zoom out and deal with our code at a higher level than “changesets”. We can then pinpoint issues of stress and zoom back in only when necessary. That means less time spent manually staring at code, though I should stress that metrics are no substitute for manual reviews.
But I Test!
A lot of people think that writing good unit tests short-circuit the need for keeping track of the code. There are a few issues with this. Firstly, it’s idealistic, since it depends on perfect test coverage, and we all know our tests are never perfect. Secondly, metrics are not meant to be your only line of defense, and there’s nothing wrong with having more than one way to verify code quality. Lastly, tracking this data helps us identify points of strain in future projects, something tests on our current system cannot help with. I’ll be talking about that in a later article, though.
Before We Begin, Some Caveats
I guess now would also be a good time to point out that not all metrics are meant to be accurate. Some metrics are accurate, but most will at best give you a ballpark figure to work with. This is not necessarily a bad thing. These estimations give you a place to start looking or find components that raise a red flag but somehow got through your code review processes. They’re not a substitute for code reviews. Metrics are mostly a science of approximation and thresholds.
As mentioned, there are many things we can track. But what “things” are most important to a software project? Let’s go over some of the more common metrics we’d want to keep track of and discuss how they can help us.
The Common Metrics
These are the really basic metrics that most of us know about:
- Lines of Code (LoC or KLoC)
- Cyclomatic Complexity (VG)
- Test Coverage (TC)
These metrics don’t really give you all that much useful information. However, they do make up a good set of building blocks for some numbers that can give us much more insight into our code. For all the Rubyists out there, you might be familiar with tools like RCov, Flog, Flay, Roodi, and the like. These tools basically check the above metrics (there’s also a metric for “duplication” in there, that one’s a little less basic).
Let’s talk about these guys:
Lines of Code (LoC or KLoC)
This is probably the hardest metric to interpret because the way we collect this information can vary greatly. We already know that the very same algorithm can have wildly different LoC counts depending on which language it is implemented in. We also know that an algorithm can be rewritten in the same language with wildly different LoC counts. This means from the outset, LoC can be an unreliable metric depending on how it is used.
There are actually a few ways to count lines of code. The simplest is to count the number of uncommented, non-whitespace lines in your source files. However, everyone but a Python programmer knows that we can stuff a lot of complexity into a single line of source, so this is not telling us the whole story. The more accurate measure of LoC (good metrics tools will do this) is to not count “lines”, but “statements”. This allows us to treat each statement as if it was on its own line (something Python programmers already expect). For example, the following Ruby code could either represent 1 LoC or 5 LoC:
10.times { result = flip_coin; tell_user(result); again = gets; break if again == "N" }
Yes, we can golf that Ruby down to 3 LoC, but that’s not something we’ll worry about. In fact, by counting statements rather than lines we can compare this value to our complexity and get a pretty useful result, but I won’t ruin the surprise. We’ll talk about that later. The point here is that there are many ways to define what a “line” is, so you should know how your tools define this metric. This might change how you can make use of the data.
As I pointed out, LoC can vary greatly, but that does not make it useless. Each codebase has its own patterns and conventions, so as long as you maintain consistency in your code, LoC should be able to give you useful information; but it will only apply to your code, not anyone else’s.
Cyclomatic Complexity (VG)
In case you're confused, Cyclomatic Complexity will be abbreviated as VG because it is abbreviated as the function v(G) in the static analysis tool McCabe IQ. Cyclomatic Complexity, for those who don't know, was a metric coined by McCabe, so v(G) is what I would consider the "official" abbreviation.
In short, cyclomatic complexity is defined as “the number of independent paths in a function”, or “the number of conditional branches + 1”. It’s a fairly easy one to calculate, though realize that languages with closures are a little harder to deal with since we can’t immediately tell if the closure is a “conditional” or “unconditional” branch. This is an important point for dynamic languages with closures (like Ruby), because it means our VG value will be a little less reliable, and we need to take that into account.
The great thing about an accurate VG measure is that it should be equal to the minimum number of tests for complete C1 code coverage. This means we can easily tell which modules are under-tested by simply comparing this value to the number of tests we have written for said module (again, ballpark, there are other factors). Note that while runtime tools like Ruby’s RCov can tell you your C1 coverage, it cannot tell you if your tests are organized properly. Mapping each code path to a separate test will give you better organized tests, and only VG can help you do this.
Note that Ruby’s “Flog” tool is not a true “Cyclomatic Complexity” measurement. It uses its own heuristics to give you a “score”, but you cannot use this number to verify against your tests.
Test Coverage (TC)
I said we would only discuss static code metrics, but I think this one deserves mentioning because it’s probably one of the most useful. TC is a runtime metric and requires a tool that can profile your tests and tell you how much of your codebase they cover (as a percentage). The Ruby tool for this is RCov as we previously mentioned. There’s not much to say here except that TC gives you a good place to start looking when trying to find under-tested components. Remember, this only gives you test coverage, not test quality, so 100% coverage is not equal to “no bugs”. Also, as mentioned in the previous section, coverage does not mean your tests are well organized. If you want to properly organize your tests, you should look at mapping each test to an individual code path, which requires the VG metric.
These are the basic metrics that most of us probably make use of daily. But as I said, these kinds of metrics are really just the building blocks for the really fun stuff, so let’s talk about those.
Better Metrics
We can get some pretty useful information out of our source code that some people might not think about tracking. Data like coupling (afferent and efferent), module stability, essential complexity, essential LoC, defects/loc, weighted methods per class, lack of cohesion of methods. Most of these metrics come from some pretty great static analysis tools such as McCabe IQ and NDepend. These tools support most static languages (plus Perl for some reason), but don’t have much support for dynamic languages like Python or Ruby. Unfortunate, but hopefully tools like these can be built for those languages. Anyway, let’s talk about these metrics.
Afferent Coupling (Ca) and Efferent Coupling (Ce)
Coupling is the measure of how much a module depends on, or is depended on by, other modules. Afferent Coupling is the measure of dependencies on a module, and Efferent Coupling is the measure of how many other modules a module depends on. This great graphic from codebetter.com should illustrate the two:
As you see, Afferent Coupling tells us how integral a module might be to the functioning of other modules. One thing you would want to make sure is that modules with a high Ca value are properly tested, as bugs in these modules are likely to have much higher impact. A high Ce value would tell you that perhaps your module has too many responsibilities and should be split up. Of course “high” here means relative to other classes in your codebase. As with most metrics, you should pay most attention to statistical outliers on a relative scale, not absolute scales.
Module Stability, or Instability (I)
Now that we’ve discussed Ca and Ce, we can talk about instability. This is where we see how these building block metrics really shine. While Ca and Ce are useful on their own, the Instability metric can give us some extra insight.
Instability is simply defined as: I = Ce / (Ca + Ce)
, or in plain English, the ratio of modules this module depends on versus the total coupling of that module. As the metric name suggests, what this tells us is how stable a module is. How does that really work? Well, if a module has many dependencies (both in and out) and most of them are outward dependencies (Ce), it’s not going to be resilient to changes in your system as a whole. On the other hand if only a small amount of the module’s total coupling are outward dependencies, it’s going to be more resilient to changes in your system.
In short, this metric can help you identify classes to double check when you plan on doing larger refactoring runs.
Essential Complexity (ev(G))
This one is specifically of interest to those of us who use dynamic languages. McCabe IQ defines this metric as:
Essential Complexity (ev(G)) is a measure of the degree to which a module contains unstructured constructs. This metric measures the degree of structuredness and the quality of the code. It is used to predict the maintenance effort and to help in the modularization process.
Unstructured constructs is a little vague, and just like a LoC metric might differ depending on the tool (and language). In Ruby, however, we would consider unstructured constructs to be things like lambdas/procs, blocks/closures and eval(). These methods would be seen as more complex and harder to test, so they should get extra attention. In static languages (and those without closures/blocks) the threshold of acceptance of this value would be a lot lower, and we would probably plan on refactoring any of the outliers.
Essential LoC (eLoC)
I don’t know of any tools that explicitly perform this metric calculation, though it is something you may have calculated informally before. Essential LoC is defined on a per method basis as eLoC = LoC / v(G)
, or in plain english, the number of statements in the method divided by the cyclomatic complexity (aka. number of code paths). This would tell us, on average, how many non-control-flow statements our methods have. A well refactored codebase would have this ratio as close to 1 as possible (even though it might have many code paths). The idea is also that methods with similar complexity should have similar LoC counts. The threshold on this would be lower than usual, but using this kind of a metric, it would be easy to identify methods that have similar complexity but take more LoC to perform the task. Outliers on a comparison of methods with equal complexity is likely to give you methods that could be refactored more easily than outliers on a pure LoC metric.
Defects Per LoC
Again, not really used formally in tools because there’s no way to automate this calculation, but this metric is pretty useful. Basically, it is the ratio of the number of reported defects over the total LoC. This data can be collected in a more granular basis, ie. per team member, per iteration, per feature. A good table showing an example can be found here. Although this one is a little more tedious to implement, it can give you some good numbers about team productivity, code quality and effectiveness. If you do Agile/Scrum, tracking these numbers is a great way to keep your team motivated on self-impovement (so long as you keep the environment positive and not overly competitive). These numbers also give you great data with which to estimate the duration of future projects. If you make your living working on many similar short-term contracts, this metric can make a big difference on your bottom line.
Weighted Methods Per Class (WMC)
WMC is basically the sum of all v(G) of a class. This is basically cyclomatic complexity as a less detailed view of your system which should make it easier to pinpoint your complex modules at a glance, rather than sifting through individual methods.
Lack of Cohesion of Methods (LCOM)
Cohesion of a module tells us the degree to which a module implements one single function; in short, the Single Responsibility Principle (SRP). LCOM, although a confusing name, tells us the opposite; the degree to which a class implements more than one function. NDepend has a great overview of this metric, but in short, lower is better, and above a certain threshold is bad.
Analyzing Metrics
Of course by doing all these calculations, with tools or not, all we get is a bunch of numbers. Just like LoC, they’re meaningless in a vacuum. Figuring out what these numbers mean is the important part of metrics.
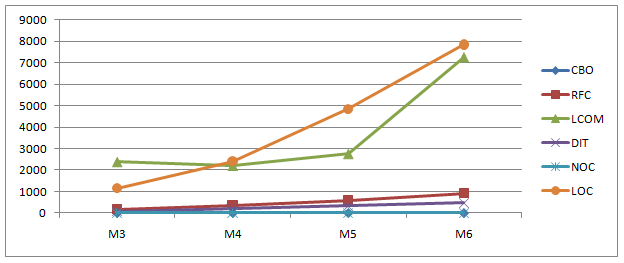
Figure 1. Keeping track of different metrics over many iterations of a project
As mentioned before, some metrics are absolute values. Metrics like cyclomatic complexity should always be below a certain value regardless of the “average” complexity of your codebase (though the actual recommendation depends on who you ask). However, most metrics, specifically those with ratios, are meant to be analyzed statistically on a relative scale in comparison to the entire codebase. Generating a boxplot is generally a good way to identify the outliers. These outliers are generally the modules/methods we want to look at because they exceed the “threshold” of normalcy in the project.
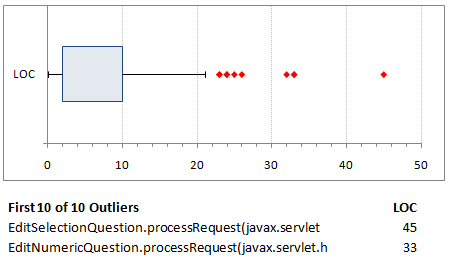
Figure 2. The box plot of LoC for methods in an application. Only outliers are shown.
Keeping track of these metrics allows us to analyze our project’s history, giving us even more statistical data to work with. We can analyze, for instance, which methods/modules change often, and we can perform meta-calculations on our data to find out the outliers in those categories. This lets us decide if, perhaps something needs to be done about those methods/modules to make them more stable. Keeping track of these metrics also lets us figure out how our team works, how effective they are and such, which lets us estimate effort for future projects of similar scope (again a future article subject).
Conclusion & Resources
Ahh, the burning question…
What Tools Should I Use?
So we talked a great deal about when and where to apply metrics, but I didn’t say anything about how to apply them. Your mileage may vary here, but there are a few tools to consider. The best ones tend to cost (a lot of) money, but here they are:
Non-Free Metrics Tools
- McCabe IQ – great tool, pretty expensive, but has some impressive metrics. Handles Java, C, C++, Perl, C#, VB, and a boat-load of older static languages.
- NDepend – Windows-only, and seems to be .NET specific. Integrated with VS.NET, but also seems to have an open source / academic license, so if you do C#/VB, this might be a good choice.
- Logiscope – Part of IBM’s Rational products. I’ve used it once before at my university and I know it supports Java, but I have no clue what else.
Free Metrics Tools
- Eclipse Metrics – Eclipse has a metrics plugin that does most of the calculations I described in the article. It likely only supports Java, though.
- Another Eclipse Metrics Plugin – Here’s another one, this one seems to be more up to date. I haven’t tried either so I can’t recommend them, and again this probably only supports Java.
- C Metrics – A list of C/C++ metrics tools. Note: most of these are extremely basic, but some of the ones at the end might be helpful.
Finally, here’s a list of metrics tools, not compiled by me, of both free and non-free metrics software. It’s a lot more extensive.
Hopefully that gives a tidy overview of some interesting metrics and perhaps gives you some ideas on how these numbers can be used in your projects. If you want some more information on metrics or are not thoroughly convinced by this post, there’s a great book titled Applying Software Metrics that you should check out.